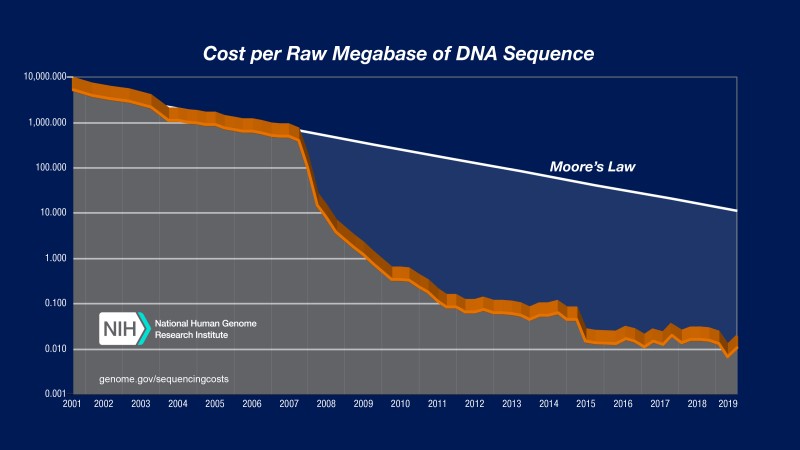
Fig 1. Sequencing cost per megabase - 2019
Bioinformatics is the study of biological data, often using a combination of mathematical, statistical and computer science techniques. Due to decreasing costs of sequencing (Fig 1), large datasets of genomic data are becoming more widely available, causing bioinformatics and computational biology to grow rapidly
Some of our most recent work in bioinformatics involves microRNAs (miRNAs). MiRNAs are small, non-coding RNA molecules that regulate gene expression. The dysregulation of miRNAs enables tumor growth, causing miRNAs to act well as cancer biomarkers. Applications of deep learning employed in the lab (Fig 2) have allowed neoplastic biopsy samples to be identified from non-neoplastic samples using miRNA sequencing data. Further work aims to increase the understanding of miRNA dysregulation by identifying similar dysregulation between different tumor types, focusing specifically on different types of neuroendocrine tumors.
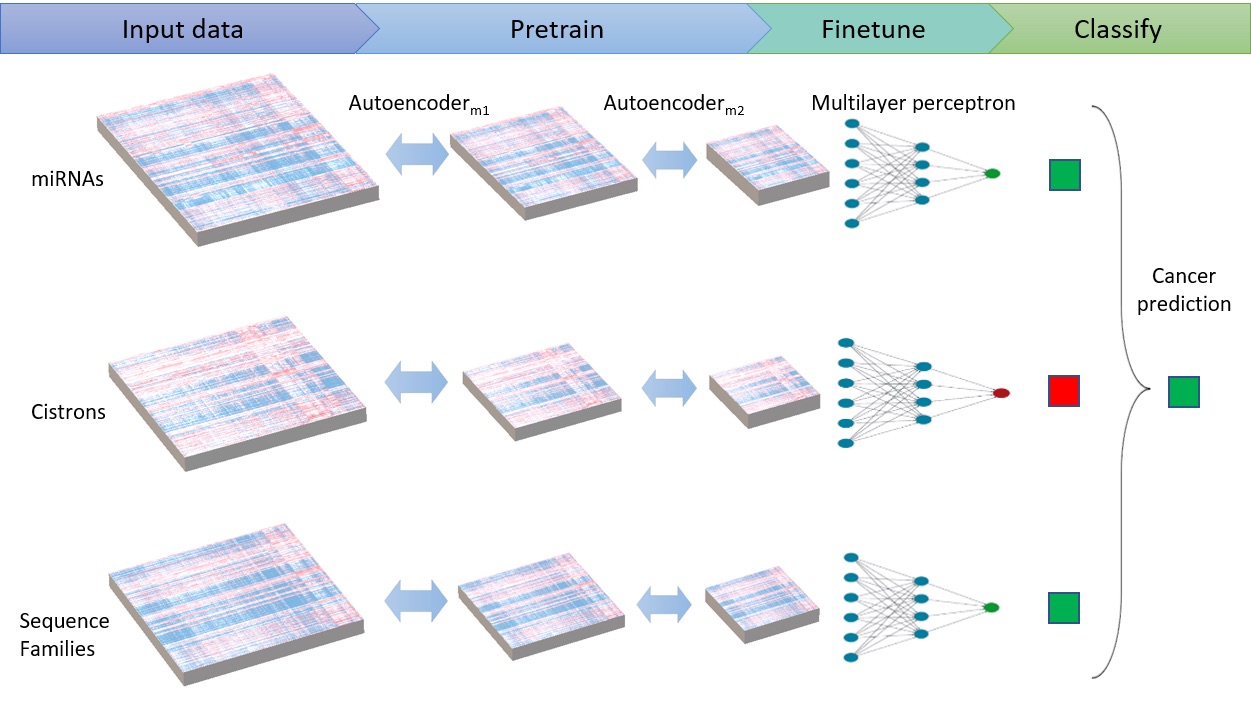
Fig 2. Deep ensemble consisting of stacked autoencoders and multilayer perceptron for cancer prediction.
Other areas that we are interested are the interactions between different genomic components. The interactions between proteins and DNA, as well as information obtained from gene, tissue, and disease association, are complex and highly reliant on each other. Visualization and increased understanding of these interactions can lead to better comprehension of disease causation and potential treatment. We have developed two Cytoscape applications, iPINBPA and iCTNet (Fig 3), which look at providing new ways of anticipating the relationship between the above-mentioned genomic components.

Fig 3. A network of five common autoimmune diseases including rheumatoid arthritis (green), type 1 diabetes (red), multiple sclerosis (yellow), Chrohn's disease (teal), and psoriasis (magenta). A) Disease-gene interaction network for five common autoimmune diseases. Each disease has unique and shared associations. RA, T1D, and MS are closely related. B) A simplified version of the network shown in A, using the "create similarity net" feature of iCTNet. In this representation, diseases are connected by edges of a color proportional to the number of shared genes. C) Same network as in A with drug-target interactions.
More specific work has been done on identifying the exact gene deformation associated with disease. Single Nucleotide Polymorphisms (SNPs) occur when an incorrect nucleotide is placed in a strand of DNA. There are millions of SNPs in each genome, most with no noticeable effects. However, some SNPs are responsible for disease; the identification of these SNPs is particularly important in understanding disease causation. Independent Component Analysis and a regression approach similar to a modified version of Fast Orthogonal Search (mFOS) are used to reduce data containing SNPs, and identify the more important deformations acting as the disease causation.